•
the Blog
Bayesian networks and causation 
By Guillaume Filion, filed under
statistics,
Bayesian networks,
causes,
correlation.
• 20 June 2015 •
The first thing you learn in statistics is that “correlation does not imply causation”. As obvious as it sounds, most human mistakes fall in this category, and not only in statistics. The major difficulty with this question is that it is fairly easy to define correlation, but it is much harder to define causation, let alone quantify it. No surprise many statisticians just avoid talking about causation to stay out of the danger zone.
However, for Judea Pearl, this is not a satisfactory answer. In his book Causality: Models, Reasoning, and Inference, he expresses his opinion vividly.
I see no greater impediment to scientific progress than the prevailing practice of focusing all our mathematical resources on probabilistic and statistical inferences while leaving causal considerations to the mercy of intuition and good judgment.
This book lays the foundation of the now popular Bayesian networks. The key idea is that you can distinguish correlation from causation if you can observe several independent causes. For instance, suppose that patients suffering from a certain type of cancer are often immunodeficient. You wonder whether immunodeficiency is a cause or a consequence of this cancer type.
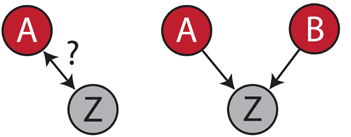
Say that variable A is whether patients have...